Applications of Graph Neural Networks (GNNs)
Unveiling the Web: Exploring Diverse Applications of Graph Neural Networks (GNNs)
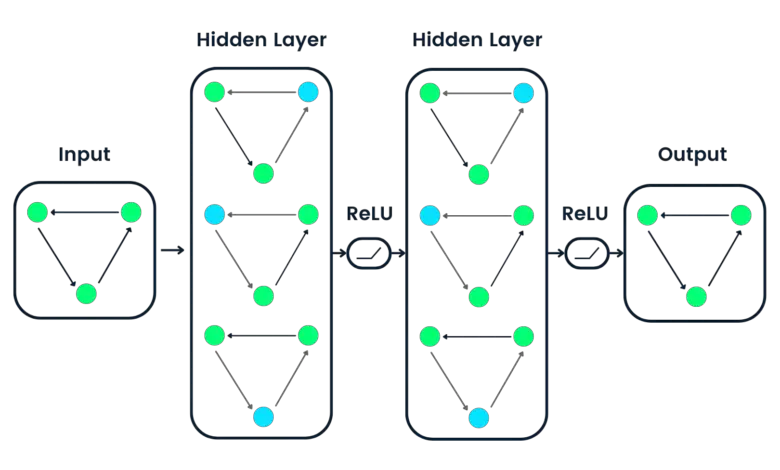
Graph Neural Networks: GNNs excel in understanding social networks by capturing relationships between individuals, predicting links, identifying influential nodes, or detecting communities.
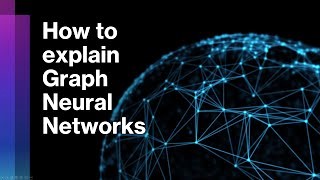
Recommendation Systems:
GNNs are used in recommendation engines to model user-item interactions, providing personalized recommendations in e-commerce, streaming platforms, or content suggestions.
Biomedical Research:
GNNs aid in analyzing molecular structures, predicting protein interactions, drug discovery, and understanding biological pathways, contributing significantly to bioinformatics and drug development.
Fraud Detection:
GNNs help detect fraudulent activities by analyzing transaction networks, identifying anomalous patterns, and flagging suspicious behavior in financial transactions or online activities.
Natural Language Processing (NLP):
GNNs are employed to analyze semantic relationships between words, sentences, or documents, enhancing tasks like document classification, sentiment analysis, and entity recognition.
Transportation and Logistics:
GNNs assist in optimizing transportation networks, predicting traffic flow, recommending routes, or optimizing delivery logistics by understanding the relationships between various nodes (e.g., roads, intersections, and traffic patterns).
Computer Vision:
GNNs have been applied to understanding spatial relationships in images, such as scene graph generation, object detection, and image segmentation tasks.
Recommendation in Knowledge Graphs:
GNNs are used in knowledge graphs to infer missing relations or properties, recommend relevant entities, and perform reasoning over graph-structured data.
Power Grid Optimization:
GNNs help model power grids, predict faults, optimize energy distribution, and improve the resilience and efficiency of electrical networks.
Criminal Network Analysis:
GNNs aid law enforcement agencies in analyzing criminal networks, identifying key players, understanding network structures, and predicting potential criminal activities