Unveiling the Power of AI in Cutting-Edge Fraud Detection
AI and fraud detection-Kat Technical
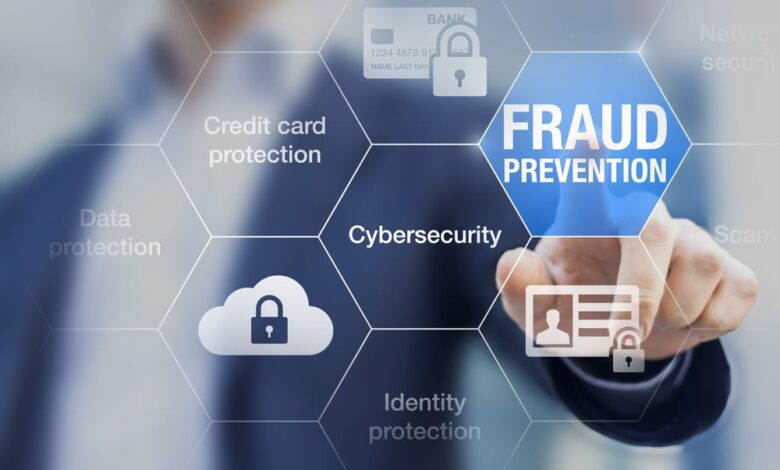
fraud detection In the realm of fraud detection, artificial intelligence (AI) stands as a beacon of hope, revolutionizing the way businesses combat fraudulent activities. By leveraging advanced machine learning algorithms and neural networks, AI-powered systems can sift through vast volumes of data with unparalleled speed and accuracy, identifying subtle patterns indicative of fraudulent behavior. With real-time detection capabilities and adaptive learning mechanisms, these systems enable businesses to stay one step ahead of fraudsters, mitigating potential losses and safeguarding their assets. As AI continues to evolve, its role in fraud detection will only become more indispensable, ushering in a new era of proactive cybersecurity measures and enhanced risk management strategies.
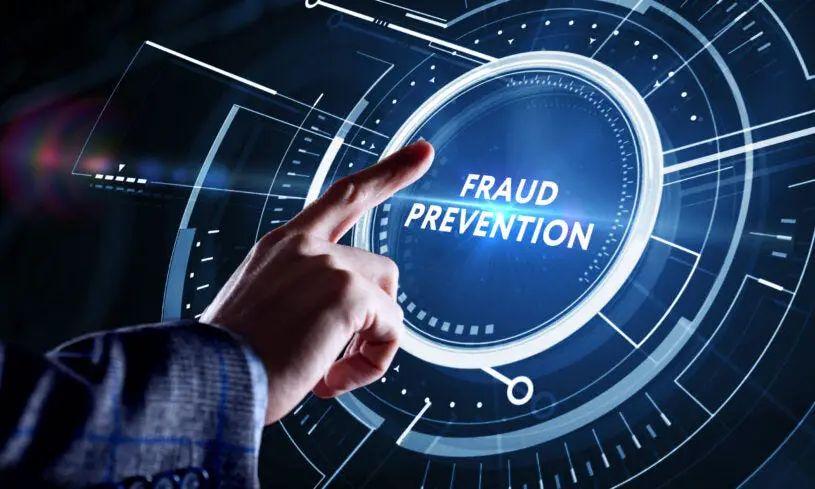
Leveraging AI for Advanced Fraud Detection
In today’s digital landscape, where online transactions have become the norm, the need for robust fraud detection mechanisms is paramount. With the increasing sophistication of fraudulent activities, traditional methods of fraud detection are proving to be inadequate. However, thanks to advancements in **Artificial Intelligence (AI)**, particularly in the realm of **machine learning**, a new era of fraud detection has emerged.
Understanding AI in Fraud Detection
AI encompasses a broad spectrum of technologies that enable machines to simulate human intelligence. In the context of fraud detection, AI algorithms are trained to analyze vast amounts of data, identify patterns, and detect anomalies indicative of fraudulent behavior. Unlike traditional rule-based systems, AI-driven fraud detection systems can adapt and evolve, continuously improving their accuracy over time.
Machine Learning Models
At the core of AI-powered fraud detection systems are **machine learning models**. These models are trained using historical data, which includes both legitimate and fraudulent transactions. Through the process of **supervised learning**, the models learn to distinguish between normal and anomalous behavior, thereby flagging suspicious activities in real time.
Neural Networks
Neural networks are a subset of machine learning algorithms that mimic the structure and function of the human brain. These networks consist of interconnected nodes, or neurons, organized into layers. By processing data through multiple layers of abstraction, neural networks can uncover intricate patterns that may elude traditional detection methods.
The Role of AI in Fraud Prevention
AI-driven fraud detection systems offer several advantages over traditional approaches:
Real-Time Detection
One of the most significant benefits of AI is its ability to detect fraud in real time. By analyzing transactions as they occur, AI algorithms can promptly identify suspicious activities and trigger alerts, allowing businesses to take immediate action to mitigate potential losses.
Enhanced Accuracy
AI-powered fraud detection systems leverage advanced algorithms that are capable of analyzing vast datasets with unparalleled speed and accuracy. By continuously learning from new data, these systems can adapt to evolving fraud tactics, ensuring high detection rates while minimizing false positives.
Adaptive Learning
Unlike static rule-based systems, AI algorithms can adapt and learn from new patterns and trends. Through a process known as **unsupervised learning**, these algorithms can detect emerging forms of fraud that may not have been previously encountered, thereby staying ahead of fraudsters.
Reduced Operational Costs
By automating the fraud detection process, AI can significantly reduce the operational costs associated with manual review processes. Moreover, AI-driven systems can handle large volumes of transactions with minimal human intervention, allowing businesses to scale their fraud detection efforts efficiently.
Implementing AI for Fraud Detection
While the benefits of AI in fraud detection are clear, implementing an effective AI-driven system requires careful planning and execution:
Data Quality and Quantity
The success of AI-driven fraud detection systems hinges on the quality and quantity of data available for training. Businesses must ensure that they have access to comprehensive datasets that accurately reflect both normal and fraudulent behaviors.
Model Training and Validation
Training AI models requires expertise in data science and machine learning. Businesses must invest in skilled professionals who can develop and fine-tune machine learning algorithms to achieve optimal performance. Additionally, rigorous validation processes are necessary to ensure that the models are accurately detecting fraud without generating excessive false positives.
Integration with Existing Systems
AI-driven fraud detection systems should seamlessly integrate with existing **risk management** and **security infrastructure**. This requires careful coordination between IT, security, and business stakeholders to ensure smooth implementation and operation.
Regulatory Compliance
Businesses operating in regulated industries must ensure that their AI-driven fraud detection systems comply with relevant regulatory requirements, such as **GDPR** and **PCI DSS**. This may involve implementing measures to protect customer privacy and sensitive data while still maintaining robust fraud detection capabilities.
The Future of AI in Fraud Detection
As AI technologies continue to evolve, the future of fraud detection looks promising. Advancements in areas such as **natural language processing**, **image recognition**, and **graph analytics** hold the potential to further enhance the effectiveness of AI-driven fraud detection systems. Moreover, the proliferation of big data and cloud computing technologies will enable businesses to leverage AI at scale, empowering them to stay one step ahead of fraudsters.
In conclusion, AI has revolutionized the field of fraud detection, offering unprecedented levels of accuracy, speed, and adaptability. By harnessing the power of AI-driven technologies, businesses can effectively combat fraud while minimizing operational costs and enhancing customer trust.